Epidemiology
This work was conducted during 2020, during the early part of the COVID-19 pandemic. Statistical analysis was performed to estimate factors such as true infections and mortality from the period when testing was limited. Also, a community model was created to look at disease spreading in communities.
COVID-19 Actual Prevalence in America Adjusted for known COVID-19 Mortality and for Excess Mortality during the Pandemic Period
Regional and temporal variation in COVID-19 test availability have limited real estimates of COVID-19 prevalence over time. Better estimates could orient EDs to plan for surges of patients. Reports of seroprevalence of immune response to COVID-19 in American communities imply between 6 and 24 fold higher rates of infection than the official RT-PCR based case counts. We used the known deaths attributed to COVID-19 and the excess deaths [4] in America during the pandemic and fit the data to reported local timed cumulative case load (immunoprevalence) to derive upper and lower estimates of the true case fatality rate for COVID-19 in America..
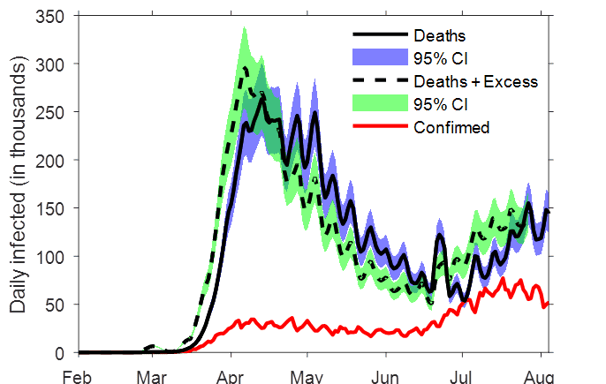
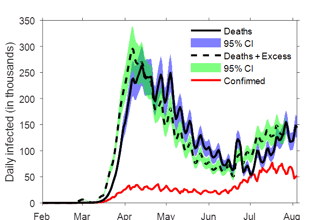
Friends, Family and Sustainability: Lessons from a Novel Model for a New COVID-19 Pandemic
SARS-CoV-2 has led to urgent and renewed interest in epidemiological modeling of novel infectious pathogens and interventions to curb them while awaiting vaccines and other effective treatments. We propose and describe a novel stochastic approach to SIR with subpopulations and ability to derive estimates of our uncertainty, both with respect to the model itself and the variables dependent on unknown characteristics of the pathogen modeled. Our model adds new value by accounting for subpopulations within a main population and for differential interactions within and between these subgroups, leading to different opportunities for infection between subgroups. We study various potential interventions during a pandemic as represented by their impact on transmission in subgroups in our model within simulations. We measure total cases over time, proportion of a populace requiring intensive care over time, and peak ICU utilization. We show convergence of our model with known models mathematically and validate our model with historical American influenza data. We then model various interventions to discern which are most effective. We find that: 1) Implementing excessively restrictive social distancing measures can have diminishing and even negative returns, particularly if these are only temporary and there are not ongoing modulated effects in effective . 2) Reducing the spread of a novel infectious disease within households and among family and friends is the most effective means by which to reduce the total infection rate. 3) To reduce the total number of infections, effective must be lowered in a sustainable fashion..
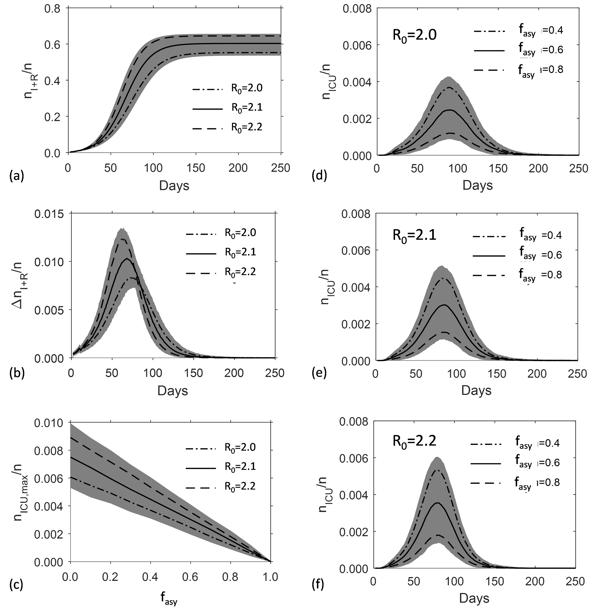
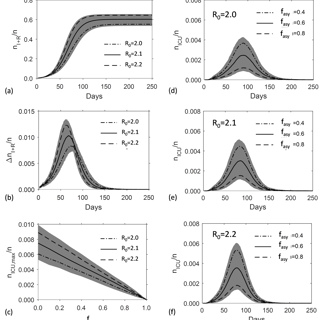